By: Parastu Basaran, Rima Boudali, Christopher Chammas, Nia Pietrobruno and Amanda Urgolo
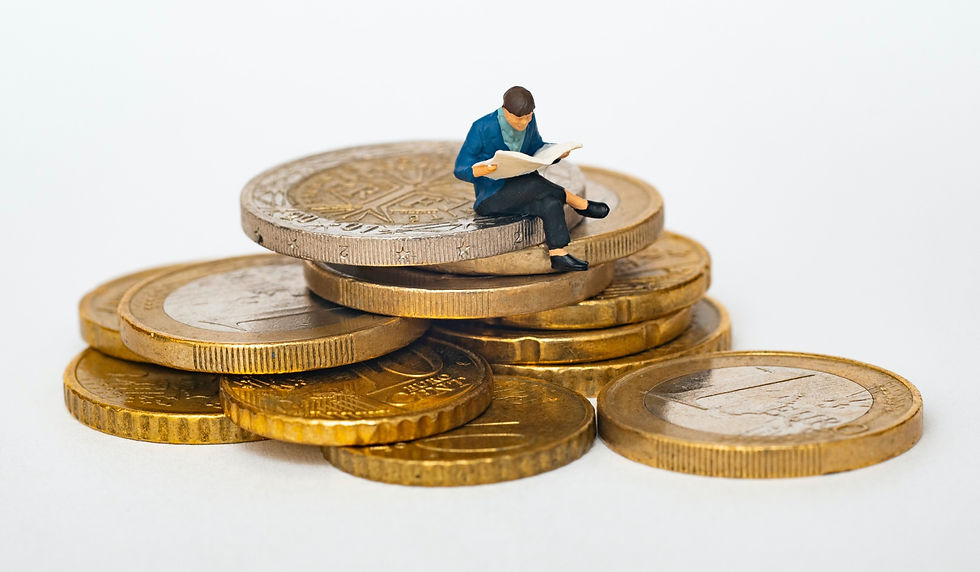
In this report, we will examine the relationship between loss aversion in situations of financial loss and gain and financial expertise. Loss aversion can be described as individuals disliking losses more than equivalent gains (Aguilar, 2021). Studies have shown that people would go to extreme lengths, including unethical behaviour, to avoid a loss. For instance, an individual would rather avoid losing $50 than gain $50 (Decision Lab, 2021). This bias is also seen in capital markets, specifically in the stock market. An individual would not purchase a seemingly risky stock even though it has the possibility of providing a higher reward (Decision Lab, 2021). To better understand this topic, it is important to note the difference between loss aversion and risk aversion. Loss aversion will consider the individual’s utility, where the pain following a loss is greater than the happiness generated by a gain. If we observe a utility function, an individual's utility is “concave over gains and convex over losses” (Bogan, 2019). For example, a $400 pay cut will result in a bigger discontent than the fulfillment of a $400 raise. Loss aversion takes into consideration the outcome whereas risk aversion does not. Risk-averse individuals prefer lower returns with known risk instead of higher returns with unknown risk (The Economic Times, 2022). In other words, risk-averse individuals dislike risk regardless of the outcome. Our topic will focus on loss aversion as we intend to experiment with variables beyond the personality of individuals.
Why Is This Topic Important?
Cognitive biases affect human decision-making daily. Whether the situation is significant or not, the fear of losing offsets the satisfaction of gaining. Loss aversion is an extremely important topic because understanding it could prevent missing out on potential gains. When people are met with a problem or opportunity, emotions may get in the way of rational thinking. For example, some people refuse to sell a stock despite contrasting market analyses, believing that a loss will not be accounted for until they sell the stock. This results in them holding the stock for a long period of time because it may never recuperate and causes them to miss out on other opportunities. Loss aversion can even cause the same person who decided to hold the losing stock to invest even more money to average down the position thinking that the money could still be recovered. This is an example of a sunk-cost fallacy (Tait & Miller, 2019). Hence, understanding loss aversion is important because once it is understood, it can be better handled and allow increased rational thinking.
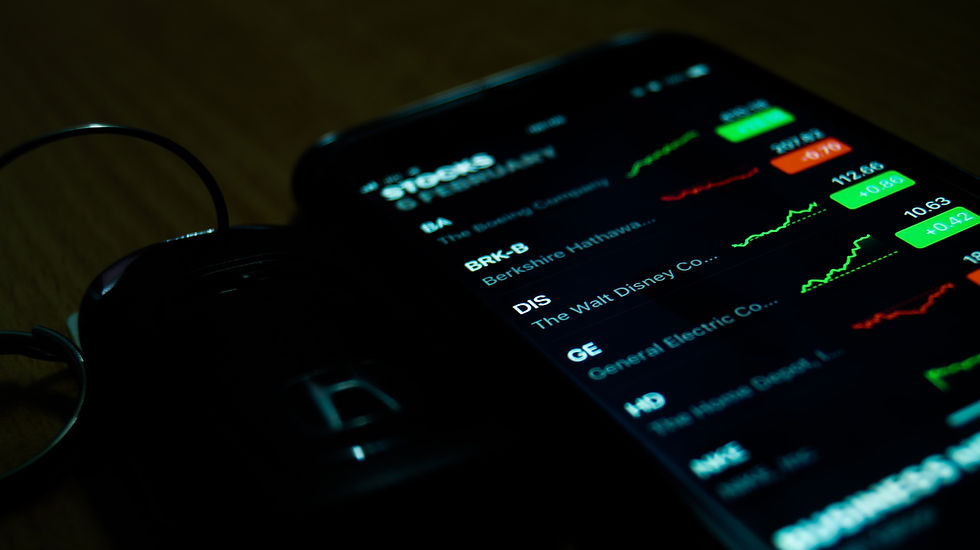
How The Issue Will Be Addressed
To determine the relationship between loss aversion and the experience and knowledge of the individual, we developed an online survey. We determined whether any of these variables affect our variable of interest—loss aversion tendencies—and to what extent. In this survey, we attempted to measure participants’ level of loss aversion in situations of financial gain or loss and gather demographic data, including age, gender, financial expertise, and cultural background, amongst others. Our hypothesis is that variables related to a participant’s financial knowledge and expertise, such as their university major, year/level of education, and perceived knowledge of finance, will have the largest effect on loss aversion tendencies in situations concerning financial gains and losses. We also posit that perceived knowledge of finance will have the greatest effect on the results.
Our Study
Sample of Subjects
The sample studied is restricted to university students in Montreal to eliminate any inconsistency. We aimed to reach an approximate sample size of 100 from a range of various backgrounds. Participants were asked their faculty of study, year of study and other typical screening questions, such as age, gender, and marital status. The gathered data falls under three different groups. The participants’ household income, country of birth and cultural background were also gathered to see if they indicated loss aversion. Hofstede’s Cultural Dimensions give us reason to believe that one’s cultural background may impact decisions regarding risks (Mooij & Hofstede, 2010).

The Experiment and Methodology
To obtain data from our pool of participants, we conducted a two-part online survey. The first part of the questionnaire pertained to demographic characteristics and experience. This information helped us categorize respondents and assess the influence of each variable on loss aversion bias. In part one, we examined independent variables (IV). These were classified into three major groups: the main IV being the Experience and Knowledge factor, and the control variables being the Wealth and Demographic factors. Categorizing control variables is credited to Filbeck et al. In the second part of the survey, we attempted to measure loss aversion, our dependent variable (DV). In each question, participants were asked to choose between two different bets presented in probabilities of gaining or losing a certain sum of money. In each pair, one bet was designed to be more attractive to loss-averse individuals. We assigned participants points for each loss-averse selection, allowing us to quantify the level of loss aversion.
To conduct this survey, we developed a list of questions to extract the desired data about our variables of interest. These questions were written in an unbiased way that prevents leading participants. We measured loss aversion using several questions to ensure that it was measured accurately. We attempted to collect information in as few questions as possible so as not to deter potential participants from completing the survey. The survey was designed to take at most 5 minutes to complete and was conducted using Google Forms. This online survey-creation platform automatically transferred response data to an Excel file for our analyses.
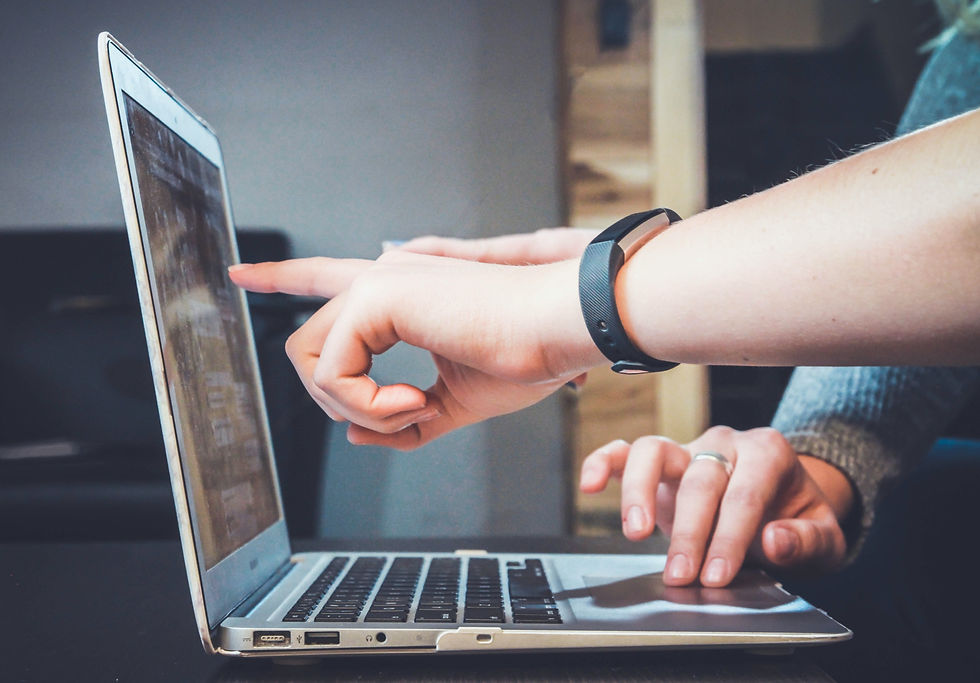
Within one week, our survey received 80 responses. In order to promote consistency within the dataset, we first ensured that each participant had answered all questions and that no responses were arbitrarily selected (e.g., always selecting option a). Then, when analyzing the second part of the survey (referring to investment tendencies), we classified participants’ answers as loss-averse or non-loss-averse. For each candidate, questions answered loss-aversely were assigned one point, and after assessing the five questions, a score over five was assigned. If the candidate had a score of three or higher, we considered that they were loss averse.
We then proceeded by creating dummy variables for the variables of interest. We created 7 dummy variables to study the impact of gender, work experience in finance, attendance of a finance 6 course, attendance of a behavioral finance course, perceived knowledge (characterized as minimal, intermediate or advanced), cultural identity and household income. Cultural identity was grouped into white and non-white, while household income was grouped into the following brackets: less than $49,999, $50,000–$99,999 and $100,000 or more. These groupings were made to ensure a simpler analysis. The dummies ranged from zero to one for most factors (binomial dummies), with the exception of perceived knowledge and household income, which were divided into three (trinomial dummies), as they comprise three different options.
After obtaining all the dummies and preparing the dataset, we imported it into Stata, a statistical software that helped us obtain relevant results. In Stata, we ran a code that is divided into four parts. The first part of the code, written with the “summarize” function, serves to provide data visualization. The second part generates the statistical significance of the means through t-tests. The third part of the code serves a similar purpose, only it is directed at the statistical significance of medians. Finally, the fourth part helps us obtain the regression equation to quantify the impact of each variable.
Results
After completing our analysis and obtaining all relevant tables, we organized them into a table to summarize our study. The table showcases what dummy was used for each variable, the number of observations in each case, the means and medians as well as the t-value showing the statistical significance of the means, and finally the regression coefficients. To remain brief, we will cover the statistical significance as it is crucial in our study, as well as the regression coefficients. We observed that all t-scores were considerably above |2.576|, entailing statistical significance to the 99% confidence level. In addition, we were able to derive relevant and reliable regression coefficients from the dataset. All coefficients are grouped in the following formula:
Loss Aversion(i) = 3.475 + (-0.3333333 ∗ GenderDummy(i)) + (-0.3618234 ∗ WorkinFinanceDummy(i)) + (-0.666666667 ∗ FinanceCourseDummy(i)) + (0.421875 ∗ BehaviouralCourseDummmy(i)) + (-0.4006923 ∗ PerceivedKnowledgeDummy(i)) + (0.1738587 ∗ CulturalDummy(i)) + (0.0.1658768 ∗ IncomeDummy(i)) + ε(i)
This formula starts with a constant of 3.475, which was obtained by regressing the loss aversion level by itself. Each of the variable coefficients was also obtained by regressing them with the loss aversion level. We found that males are less loss-averse than females by a factor of –0.333, people that have worked in finance are less loss-averse than those who have not by a factor of –0.362, people who have taken a finance course are less loss-averse than people who have not by a factor of –0.667, people that have taken a behavioral finance course are more loss-averse than people who have not by a factor of +0.422, and people with higher perceived knowledge of finance are less loss-averse by a factor of –0.401. Dummies that take on a value of two within the equation could be considered as an increase in intensity of the factor, but further research would be needed to confirm the exact level of increase in the factor.
Interestingly, we see that all this data helps us understand what may drive loss aversion levels within people, which we describe further within the conclusion.

Conclusions
Our survey and analysis determined that several demographic and personal factors are correlated to an individual’s exhibited loss-aversion. Notably, completion of a finance course was the factor with the highest correlation. The second and third highest correlations were assigned to completion of a behavioral finance course and perceived financial knowledge, respectively. Meanwhile, the demographic characteristics, such as culture and income, proved to have a smaller impact on loss aversion. As such, we assess that our hypothesis is supported; factors relating to a participant’s financial knowledge and expertise have the largest impact on their loss aversion tendencies.
To better explore our results, we investigated potential causes for the identified trends. It is possible that men are less loss-averse due to evolutionary differences, as described by Pawlowski et al. (2008). We also posit that individuals with knowledge of investment, whether through a course or work experience, are more willing to take risks because they can understand whether and to what degree potential gains outweigh potential losses. Meanwhile, inexperienced students may be more hesitant to take risks if they cannot evaluate the outcomes.
We also found that in three out of the five questions designed to assess loss-aversion, between 70% and 80% of participants selected the more loss-averse choice. However, in two 8 questions, participants were more evenly split between the loss-averse and non-loss-averse options. Questions 15 and 1 differed from the others in terms of scale. Both offered the choice between no loss or a loss of $30 and $100, respectively. Individuals may be more willing to take risks with smaller sums of money. Additionally, the responses to these questions demonstrate that individuals are less likely to be loss-averse if they have the option to choose between a loss and no loss. In questions that offered a choice between a small loss and a large loss, the loss-averse option was largely selected.
Limitations and Rationale
We acknowledge that some limitations exist in our research, including the constraint of time, the sample size, and the student body and methods of analysis. For instance, 62 out of 80 (77.6%) of those surveyed were enrolled in the Faculty of Business, 95% were single and 79% were between the ages of 20 and 24. This makes it difficult and inaccurate to draw conclusions from these variables as the sample might not be diverse enough.
With regards to methods of analysis, questions with 6 or more options to choose from might make running a regression more difficult, and less statistically significant as the number of observations may be too small to draw any significant conclusions. This resulted in the grouping of answers, and an example of this can be seen within the “household income” question. We also did not run interactive dummies because of the size restriction and time restriction of our report. However, we acknowledge that these different methods might lead to different results. Lastly, we acknowledge that though some results show significant correlation, we cannot draw unequivocal conclusions as to “why” this correlation exists.
Next Steps
We can now confirm that several variables may come into play when trying to assess the individuals’ loss aversion. The next step that would allow us to further develop our experiment would be looking at mixing variables within regressions and seeing how the coefficients are affected (interactive dummies). Another step would be to use our survey on a new sample of full-time employees. We would then have the chance to compare and see if some correlations still apply.
References
Aguilar, O. (2021, November 3). Fundamentals of Behavioral Finance: Loss aversion bias. Schwab Brokerage. Retrieved February 6, 2022, from https://www.schwabassetmanagement.com/content/loss-aversion-bias
Bogan, V. (2019, February). Risk Aversion vs. Loss Aversion: What is the Big Difference? Retrieved March 31, 2022, from https://www.hartfordfunds.com/insights/investorinsight/risk-aversion-vs-loss-aversion.html
De Mooij, M. & Hofstede, G. (2010). The Hofstede model, International Journal of Advertising, 29:1, 85-110, DOI: 10.2501/S026504870920104X
Filbeck, G., Hatfield, P., & Horvath, P. (2005). Risk Aversion and Personality Type. Journal of Behavioral Finance, 6(4), 170–180. https://doi.org/10.1207/s15427579jpfm0604_1
Loss aversion - biases & heuristics. The Decision Lab. (2021, September 30). Retrieved February 6, 2022, from https://thedecisionlab.com/biases/loss-aversion/
Pawlowski, B., Atwal, R. & Dunbar, R.I.M. (2008). Sex Differences in Everyday Risk-Taking Behavior in Humans. Journal of Evolutionary Psychology, 6(1): 29-42. Retrieved from https://journals.sagepub.com/doi/pdf/10.1177/147470490800600104#:~:text=We%20h ave%20shown%20that%20males,feature%20of%20human%20male%20psychology.
Tait, V., & Miller, Jr., H.L. (2019). Loss Aversion as a Potential Factor in the Sunk-Cost Fallacy. International Journal of Psychological Research, 12(2),8-16. Retrieved from https://www.redalyc.org/articulo.oa?id=299062446002
The Economic Times. (2022). Definition of 'Risk Averse'. Retrieved March 31, 2022, from https://economictimes.indiatimes.com/definition/risk-averse
Comments